Factor analysis is based on idea that an asset’s returns can be predicted using relationship between the asset’s expected return and a number of variables that capture systematic risk. Those variables are called factors and they cover company’s financials, trends, macroeconomic conditions, etc.
Factor analysis is a useful tool to identify securities that may be temporarily mis-priced, before the market eventually corrects and securities move back to their fair value.
Predicting prices is hard
No one can predict future, unless you have a crystal ball. Predicting prices is notoriously difficult, especially for longer time frames. Investing based on factor analysis is taking different approach.
The main claim here is that there is an intrinsic characteristic that drives the returns of assets and assets classes. Let’s explore very simplified example. When interest rates are low, market is optimistic and goes up. When interest rates rises, market gets volatile.
Let say we have company A with high operating expenses and company B with with good profitability, low debt, low operating expenses. When interests rates are low and cash is relatively cheap, it’s still possible to support growth of company A by fuelling additional money into the business. It might be seen as better potential for growth than company B.
However, when situation turns and interest rates rises, companies with good profitability will do relatively better than others. Company A will struggle to support additional growth, while B will do just fine. That’s because profitability plays a big role in such economic situation and company B is better positioned for such situation.
Factors are those driving forces behind the market returns and they explain why company B is doing better than company A. Note that company’s profitability is just one of the factors that play role in determining company’s returns.
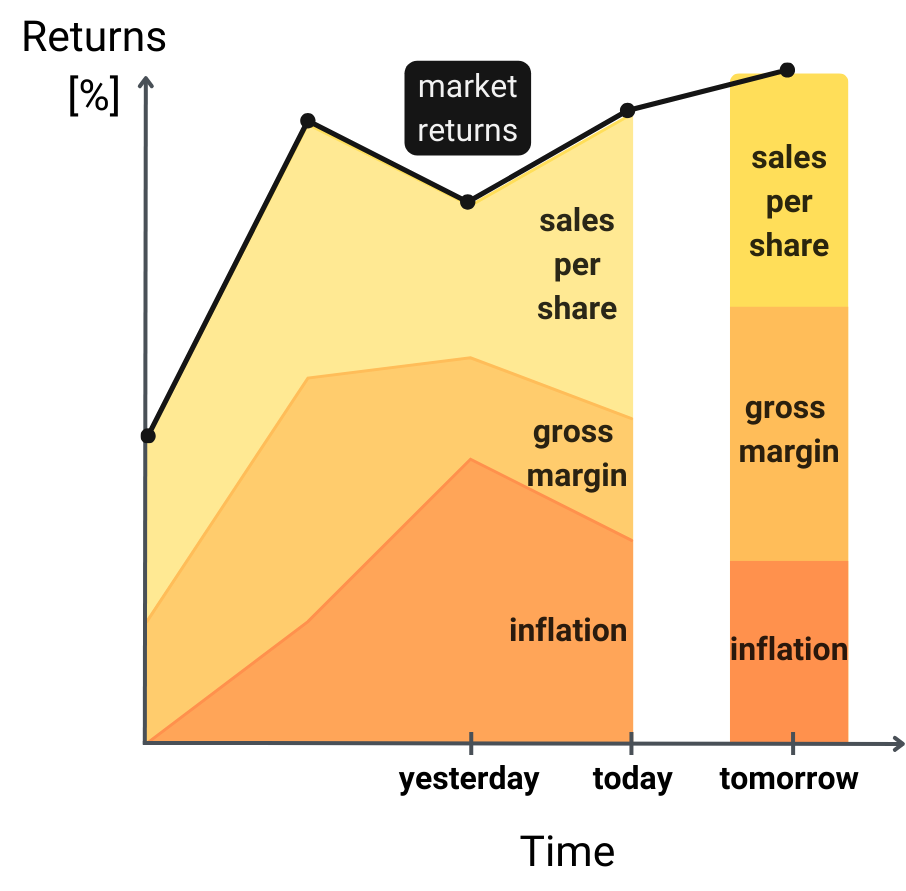
So the overall market return can be split into set of sub returns attributed to particular factors. And if we can discover and quantify those factors that play a major role on the current market, we can identify companies which will be doing better than others. The main task of factor analysis is to determine market factor composition and factor loading of all the companies.
Factor loading
The factor analysis has it’s roots in what is known as the arbitrage pricing theory. The arbitrage pricing model (APM) attempts to explain the relationship between an asset’s expected return and its risk characteristics. It suggests that the expected return of an asset can be determined by its exposure to various factors that influence its price. Based on the theory, researchers perform factor analysis to identify a set of factors, that explain the so-called “cross-section” of returns, or the distribution of returns at a given point in time. These factors can include macroeconomic indicators (e.g., interest rates, inflation), industry-specific variables (e.g., oil prices for energy companies), or company-specific factors (e.g., earnings growth).
A portfolio with a high factor loading in addition to market beta can be expected to earn a higher return than a portfolio with exposure to market beta alone.
Moreover, these factors are generally independent and uncorrelated with one another. Combining exposure to multiple factors can actually decrease the volatility of a portfolio even while increasing the return.
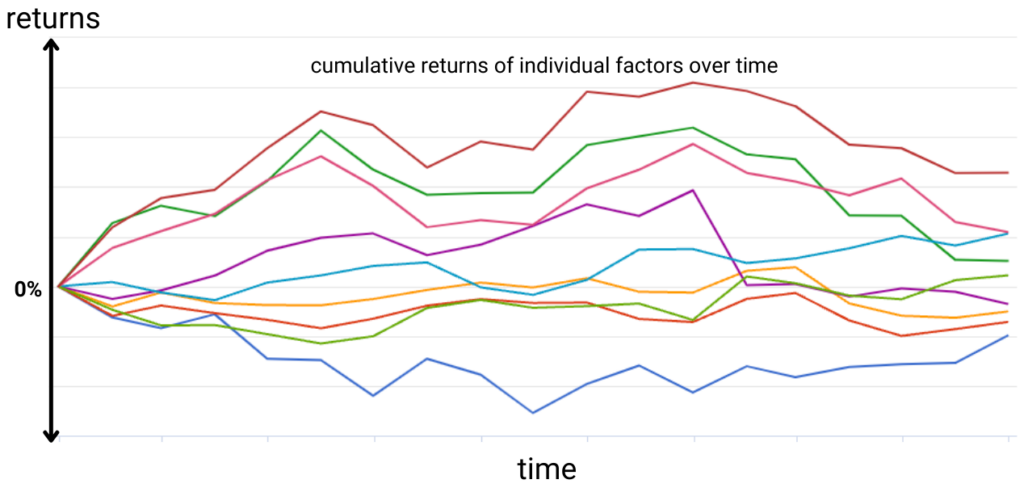
Portfolio of uncorrelated assets that perform poorly isn’t worth much. When running an analysis, it’s important to find how have they performed over time, how consistent they are, how reliable etc. In fact there are number of consideration need to be taken into account while working with factors.
Factor analysis
The goal is to find factors, that are uncorrelated with market beta and with each other, ideally some of them are also negatively correlated with one another.
Factors represent the holy grail of portfolio diversification.
If we can find 15 or more such returns streams, we can greatly improve our return to risk ratio compared to just taking whole market risk in form of buying an ETF.
That itself is a perfect task for AI, thanks to the ability to process large amount of data and performing complex computations. On top of the main purpose for factor analysis the AI approach also helps to solve some of the big challenges with factor investing.
Factor analysis challenges
Defining a factor is hard
There are over 10 thousand factors available and new ones are being created every day by researchers and economists. There are a number of “value” indices and value factor funds. Each of them might be constructed differently, using different characteristics, different weights and each of them might define value in slightly different way. Here are examples of value strategies derived from different factors and their weights. All strategies are valid, all can be labeled as “value” and yet they performed very differently over the years.
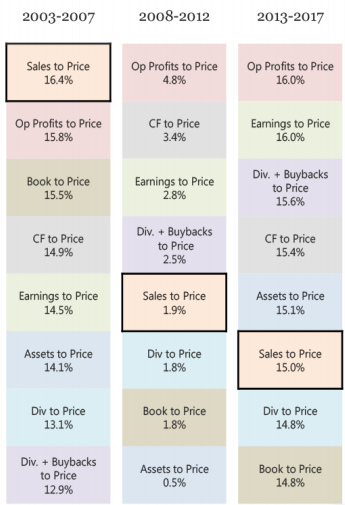
Factors can change
The traditional, academic definition of value was the P/B ratio, but many people now think that P/B is an outdated measure of value. Book value excludes brand, intellectual property, customer loyalty, data all of which are cornerstones of most of today’s service companies. Factors changing may cause problems for funds who might be slow in updating their own factor definitions in light of new evidence.
Factors decay over time
There’s considerable evidence showing that factor returns reduce after the factor becomes widely known. The more people know about a factor, the more people try to exploit it. After the factors became known, the actual returns were much lower than was predicted.
It’s difficult to determine when a factor breaks down
It’s very difficult to tell when a factor broke down as it would still occasionally behave as if it worked. To make matters worse, it’s not in the interests of financial institutions to disprove the efficacy of a strategy that their clients are invested in. All of this could lead to investors maintaining positions in factors which no longer work.
Multi factor analysis powered by AI
The aforementioned issues with factor investing suggest, that single factor doesn’t perform well under all market conditions. That’s why we have been working on machine learning models, able to find current relevant factors. The AI system then helps to rank the companies based on those factors and to build well diversified portfolio.
It’s very useful to label factors as “value”, “growth” etc. It helps investors to understand what is going on with the market and the portfolio. However each month the particular factor behind label “value” might be different. That’s because different market conditions might be better explained by different factors. It is also crucial to quickly react to changes and rebalance portfolio whenever company score changes.
The unmatched performance of AI proved to play crucial role in maintaining strategy profitable in various market conditions. It also helped to solve the issues of multi-factor portfolios. It allowed us to:
- re-evaluate the most significant factors every day
- quickly react to changing market conditions
- disregard those factors that ceased to exists or their predictive power became insignificant.
If you are interested in using factor analysis in your investment strategies, don’t hesitate to contact-us. At Analytical platform, we can deliver current market’s factor loading on regular basis.